Research
The work of the group lies on the
interface between statistical mechanics and quantitative biology.
At the centre is the relationship between fluctuations and
noise in biological systems, the corresponding statistical ensembles,
and biological function. This connection emerges at very different
levels and timescales, from stochastic modeling of gene expression to
the evolution of regulatory DNA. Our current research focus is on
cancer, especially the emergence of therapy resistance.
The emergence of resistance to targeted cancer therapy
|
Molecularly targeted cancer therapy can lead to rapid regression of
a tumour. However, in most cases
this regression is followed by the rapid expansion
of therapy-resistant mutants. In an collaboration with researchers at the Department of Translational Medicine
(Sos and Thomas labs, University Clinic Cologne), we have explored the spectrum of
mutations conferring resistance to therapy in a cell-line model of
lung cancer (PC-9 cells). This has lead to drug combinations which
inhibits all resistant mutants found in a population of tumour cells
which is large enough be detected in a clinical setting. Based on
these results, we are currently exploring dosing
schemes using the predicted evolution of the resistant clones.
|
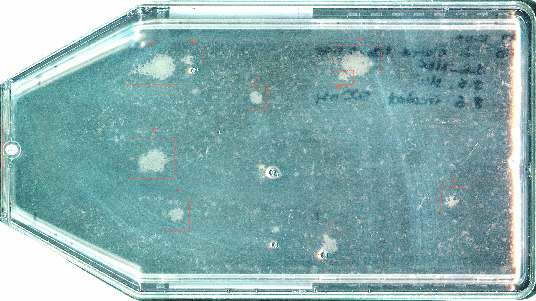
Resistant clones emerging in a population of PC-9 cells after 3 weeks
of treatment with the EGFR-inhibitor erlotinib. In [1] we
characterize and target the different resistance mechanisms found in a
population corresponding in size to a tumour of 1cm diameter.
|
Stochastic dynamics of gene expression
|
Gene transcription takes place out of equilibrium, with the rate of expression of a
gene constantly changing due to the presence or absence of specific
proteins called transcription factors. Random fluctuations of
molecular concentrations can have large effects on cells, such as
transitions between metastable states. In a collaboration with
experimentalists of the
Veening lab (Groningen), we have pinpointed the fluctuation causing
the transition to the induced state of the paradigmatic lactose-uptake pathway in
E. coli and developed a statistical theory of this
transition [1]. Past work was on
out-of-equilibrium properties of gene expression [2], the determination of
regulatory interactions between genes from gene expression time
series [3], and fluctuations in the dynamics of non-coding RNA [4].
[1] |
What makes the lac-pathway switch: identifying the fluctuations that
trigger phenotype switching in gene regulatory systems
P. Bhogale, R. Sorg, J.-W. Veening, J. Berg,
Nucleic Acids Research; doi: 10.1093/nar/gku839 (2014)
| [2] |
Out-of-equilibrium dynamics of gene expression and the Jarzynski equality,
J. Berg, Phys.Rev.Lett 100, 188101 (2008)
| [3] |
Dynamics of gene expression and the regulatory inference problem,
J. Berg, Europhys. Lett. 82, 28010 (2008)
| [4] |
Quantitative analysis of competition in post-transcriptional regulation reveals a novel signature in target expression variation,
F. Klironomos and J. Berg, Biophysical Journal, 104 (4), 951-958 (2013)
|
|
The rate at which the lac-pathway switches to the induced state (log-scale)
against the inverse of the inducer concentration. Our model
predicts a simple relationship that is borne out very well
experimentally (orange triangles), see [1].
|
Statistical mechanics, inverse problems, and inference
|
We study pure and applied problems at the interface between
statistical mechanics and machine learning. An example is the
inverse Ising problem: how to reconstruct couplings and fields of an
Ising model given observables such as spin-spin correlations. We
contributed a novel method based on the Bethe approximation, which is
exact on tree-like lattices [1], and extended mean-field approaches to
both ferromagnetic and glassy low-temperature phases of Ising models [2].
[1] |
Inverse statistical problems: from the inverse Ising problem to data science,
H.C. Nguyen, R. Zecchina, and J. Berg, Advances
in Physics,
66 (3), 197-261 (2017).
| [2] |
Mean-field theory for the inverse Ising problem at low temperatures,
H.C. Nguyen and J. Berg, Phys. Rev. Lett. 109, 050602 (2012).
| [3] |
Bethe-Peierls approximation and the inverse Ising problem,
H.C. Nguyen and J. Berg, J. Stat. Mech., P03004 (2012).
| [4] |
Significance analysis and statistical mechanics: an application to
clustering,
M. Łuksza, M. Lässig and J. Berg, Phys. Rev. Lett.
105, 220601 (2010).
|
|
Reconstruction error at different temperatures for model with a glassy
low-T phase (SK-model) against inverse temperature and for different
numbers of thermodynamic states. See [2] for details.
|
Statistical mechanics of bio-molecular networks
|
We have developed an equilibrium statistical
mechanics of networks with local connectivity correlations
[1], which links a network Hamiltonian with the corresponding
statistical observables. This statistical approach forms the
theoretical basis for identifying functional units in biological
networks. Examples are repeated patterns in networks, called network
motifs, which can be identified using Bayesian inference on models of
correlations within networks [2]. The evolutionary dynamics of
networks of related species can be traced by graph alignment, which is
based on a stochastic evolution model for biological interactions and
for DNA sequences [3,4]. We have used this approach to analyze
coexpression networks of human and mouse, and the protein interaction
networks of different herpesviruses. A software package called GraphAlignment
is available for download.
[1] |
Correlated random networks,
J. Berg and M. Lässig,
Phys. Rev. Lett. 89 (22), 228701 (2002).
| [2] |
Local graph alignment and motif search in biological networks,
J. Berg and M. Lässig,
PNAS 101(41), 14689-14694 (2004).
| [3] |
Cross-species analysis of biological networks by Bayesian alignment,
J. Berg and M. Lässig,
PNAS 103 (29), 10967-10972 (2006).
| [4] |
From protein interactions to functional annotation: Graph alignment in
Herpes,
M. Kolář, M. Lässig, and J. Berg,
BMC Systems Biology, 2:90 (2008).
|
|
Alignment of the protein interaction networks of two different Herpes
viruses [4]. Visualisation by Jörn Meier.
|
Back