Modern high-throughput experiments generate datasets of molecular interactions at the systems level, for example protein interaction networks and expression arrays. Our work on networks has focused on the identification of structural elements that may point to functional units (such as clusters and motifs) and on global similarity analysis by alignment of networks. An important part is to develop stochastic models of networks and their evolution.
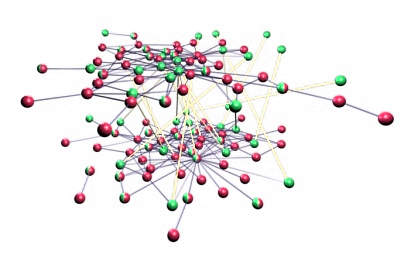
An alignment showing structural similarities between networks.
Significance analysis and statistical mechanics: an application to clustering
M. Luksza, M. Lässig, and J. Berg, Phys. Rev. Lett. 105, 220601 (4 pages), (2010)
This paper addresses the statistical significance of structures in random data: Given a set of vectors and a measure of mutual similarity, how likely does a subset of these vectors form a cluster with enhanced similarity among its elements? The computation of this cluster p-value for randomly distributed vectors is mapped onto a well-defined problem of statistical mechanics. We solve this problem analytically, establishing a connection between the physics of quenched disorder and multiple testing statistics in clustering and related problems. In an application to gene expression data, we find a remarkable link between the statistical significance of a cluster and the functional relationships between its genes.
Bayesian analysis of biological networks: Clusters, motifs, cross-species correlations
J. Berg and M. Lässig, in Statistical and evolutionary analysis of biological networks, ed. M.P.H. Stumpf and C. Wiuf, Imperial College Press, London (2009)
An important part of the analysis of bio-molecular networks is to detect different functional units. Different functions are reflected in a different evolutionary dynamics, and hence in different statistical characteristics of network parts. In this sense, the
global statistics of a biological network, e.g., its connectivity distribution, provides a background, and
local deviations from this background signal functional units. In the computational analysis of biological networks, we thus typically have to discriminate between different statistical models governing different parts of the dataset. The nature of these models depends on the biological question asked. We illustrate this rationale here with three examples: identification of functional parts as highly connected
network clusters, finding
network motifs, which occur in a similar form at different places in the network, and the analysis of
cross-species network correlations, which reflect evolutionary dynamics between species.
From protein interactions to functional annotation: graph alignment in Herpes
M. Kolar, Lässig, and J. Berg, BMC Syst Biol. 2, 90, (2008)
Background: Sequence alignment is a prolific basis of functional annotation, but remains a challenging problem in the 'twilight zone' of high sequence divergence or short gene length. Here we demonstrate how information on gene interactions can help to resolve ambiguous sequence alignments. We compare two distant Herpes viruses by constructing a graph alignment, which is based jointly on the similarity of their protein interaction networks and on sequence similarity. This hybrid method provides functional associations between proteins of the two organisms that cannot be obtained from sequence or interaction data alone.
Results: We find proteins where interaction similarity and sequence similarity are individually weak, but together provide significant evidence of orthology. There are also proteins with high interaction similarity but without any detectable sequence similarity, providing evidence of functional association beyond sequence homology. The functional predictions derived from our alignment are consistent with genomic position and gene expression data.
Conclusion: Our approach shows that evolutionary conservation is a powerful filter to make protein interaction data informative about functional similarities between the interacting proteins, and it establishes graph alignment as a powerful tool for the comparative analysis of data from highly diverged species.
Cross-species analysis of biological networks by Bayesian alignment
J. Berg and M. Lässig, Proc. Natl. Acad. Sci. 103, 10967, (2006)
Complex interactions between genes or proteins contribute a substantial part to phenotypic evolution. Here we develop an evolutionarily grounded method for the cross-species analysis of interaction networks by alignment, which maps bona fide functional relationships between genes in different organisms. Network alignment is based on a scoring function measuring mutual similarities between networks, taking into account their interaction patterns as well as sequence similarities between their nodes. High-scoring alignments and optimal alignment parameters are inferred by a systematic Bayesian analysis. We apply this method to analyze the evolution of coexpression networks between humans and mice. We find evidence for significant conservation of gene expression clusters and give network-based predictions of gene function. We discuss examples where cross-species functional relationships between genes do not concur with sequence similarity.
Structure and evolution of protein networks: a statistical model for link dynamics and gene duplications
J. Berg, M. Lässig, and A. Wagner, BMC Evol. Biol. 4, 51, (2004)
Background
The structure of molecular networks derives from dynamical processes on evolutionary time scales. For protein interaction networks, global statistical features of their structure can now be inferred consistently from several large-throughput datasets. Understanding the underlying evolutionary dynamics is crucial for discerning random parts of the network from biologically important properties shaped by natural selection.
Results
We present a detailed statistical analysis of the protein interactions in Saccharomyces cerevisiae based on several large-throughput datasets. Protein pairs resulting from gene duplications are used as tracers into the evolutionary past of the network. From this analysis, we infer rate estimates for two key evolutionary processes shaping the network: (i) gene duplications and (ii) gain and loss of interactions through mutations in existing proteins, which are referred to as link dynamics. Importantly, the link dynamics is asymmetric, i.e., the evolutionary steps are mutations in just one of the binding parters. The link turnover is shown to be much faster than gene duplications. Both processes are assembled into an empirically grounded, quantitative model for the evolution of protein interaction networks.
Conclusions
According to this model, the link dynamics is the dominant evolutionary force shaping the statistical structure of the network, while the slower gene duplication dynamics mainly affects its size. Specifically, the model predicts (i) a broad distribution of the connectivities (i.e., the number of binding partners of a protein) and (ii) correlations between the connectivities of interacting proteins, a specific consequence of the asymmetry of the link dynamics. Both features have been observed in the protein interaction network of S. cerevisiae.
Local graph alignment and motif search in biological networks
J. Berg and M. Lässig, Proc. Natl. Acad. Sci. 101, 14689, (2004)
Interaction networks are of central importance in postgenomic molecular biology, with increasing amounts of data becoming available by high-throughput methods. Examples are gene regulatory networks or protein interaction maps. The main challenge in the analysis of these data is to read off biological functions from the topology of the network. Topological motifs, i.e., patterns occurring repeatedly at different positions in the network, have recently been identified as basic modules of molecular information processing. In this article, we discuss motifs derived from families of mutually similar but not necessarily identical patterns. We establish a statistical model for the occurrence of such motifs, from which we derive a scoring function for their statistical significance. Based on this scoring function, we develop a search algorithm for topological motifs called graph alignment, a procedure with some analogies to sequence alignment. The algorithm is applied to the gene regulation network of Escherichia coli.
Correlated Random Networks
J. Berg and M. Lässig, Phys. Rev. Lett. 89, 228701, (2002)
We develop a statistical theory of networks. A network is a set of vertices and links given by its adjacency matrix c, and the relevant statistical ensembles are defined in terms of a partition function Z=Σexp([-βH(c)]. The simplest cases are uncorrelated random networks such as the well-known Erdös-Rényi graphs. Here we study more general interactions H(c) which lead to correlations, for example, between the connectivities of adjacent vertices. In particular, such correlations occur in optimized networks described by partition functions in the limit β --> infinity. They are argued to be a crucial signature of evolutionary design in biological networks.
go back 